As customers, we all want to feel unique, heard, and understood in our interactions with brands. The level of personalisation provided by digital products we use daily, like YouTube, Spotify, Amazon, or Instagram, has set a high standard. Personalisation has become so essential that when we encounter irrelevant content, ads, or products, it can be irritating and frustrating. For instance, imagine living in a city apartment and receiving emails or ads about gardening products. You'd likely wonder, "What does this have to do with me? Why am I receiving this?"
.webp)
We all recognise what "good" looks like from a consumer's perspective. However, achieving this operationally is a complex endeavour. This is where generative AI (GenAI) comes into play. GenAI is revolutionising marketing and customer experience. Leveraging advanced machine learning algorithms empowers businesses to deliver truly personalised content and experiences at an unprecedented scale, offering a more cost-effective and time-efficient approach.
Generative AI vs Ruled-Based AI
Before we discuss how to achieve 1-to-1 personalisation at scale, let's examine the basic differences between ruled-based AI and GenAI in the context of personalisation.
- Adaptability: GenAI models can adapt to new data and continuously improve their outputs without explicit rule updates.
- Creativity and Innovation: GenAI can generate original content such as text, images, music, and designs from existing data, whereas rule-based systems are limited to predefined rules.
- Pattern Creation: Generative AI excels at pattern creation, whereas rule-based systems are better at pattern recognition.
- Unsupervised Learning: GenAI uses unsupervised or self-supervised learning to identify patterns and relationships without explicit labels, whereas rule-based systems rely on predefined rules.
- Data Augmentation: Generative AI easily creates synthetic data to augment existing datasets, which is useful in data-scarce environments.
- Personalisation: Generative AI gives users personalised experiences by crafting content adapted to individual preferences, whereas rule-based systems offer standardised responses.
- Transparency: Rule-based systems are generally more transparent and interpretable compared to generative models, which can be opaque due to the complexity of their inner workings.
- Ethical Concerns: Generative AI can be misused to generate deep-fake content and other potentially harmful outputs fueled by the ethical challenges of misuse and misinformation.
- Quality Control: Ensuring the generated content meets quality standards can be challenging, especially since generative AI is notorious for generating false information.
How can GenAI enable 1-to-1 personalisation at scale?
Generative AI systems, such as Large Language Models (LLMs), can analyse vast customer data to uncover deep insights about individual preferences, behaviours, and needs. Unlike traditional rule-based personalisation approaches, GenAI can dynamically generate unique content tailored to each customer's profile.
Consider the example of a fashion retailer using generative AI to craft personalised product recommendations and marketing messages. By ingesting data on a customer's past purchases, browsing history, demographic information, and even psychographic traits, the AI can generate custom product descriptions, outfit suggestions, and promotional copy that speak directly to that individual's style and interests. This level of personalisation goes far beyond simplistic segmentation or one-size-fits-all recommendations.
GenAI can produce content that feels as if it was crafted by a human marketing expert for a single customer, not a generic template applied to an entire audience segment. By delivering these highly relevant experiences at scale, businesses can drive dramatic improvements in customer satisfaction, loyalty, and revenue.
.webp)
What data is needed to train effective personalisation models?
The key to unlocking the full potential of GenAI for personalisation lies in the quality and breadth of the underlying data. Effective personalisation models require a comprehensive view of each customer, drawing from both first-party and third-party data sources.
First-party data, such as purchase history, browsing behaviour, and customer profile information, provides the foundation for understanding individual preferences and behaviours. This data is typically collected directly from the brand's own touchpoints and customer interactions.
Third-party data sources, such as demographic data, lifestyle information, and social media activity, can offer insights into broader consumer trends, psychographics, and market dynamics.
By blending these diverse data streams, businesses can develop a more holistic and accurate view of each customer. However, it's crucial to navigate the ethical considerations around data usage. Customers are increasingly aware of privacy concerns and expect brands to handle their personal information responsibly. Strict data governance policies, transparent data collection practices, and robust security measures are essential to building trust and maintaining the ethical integrity of personalisation efforts.
Let's dive more into the ethical implications.
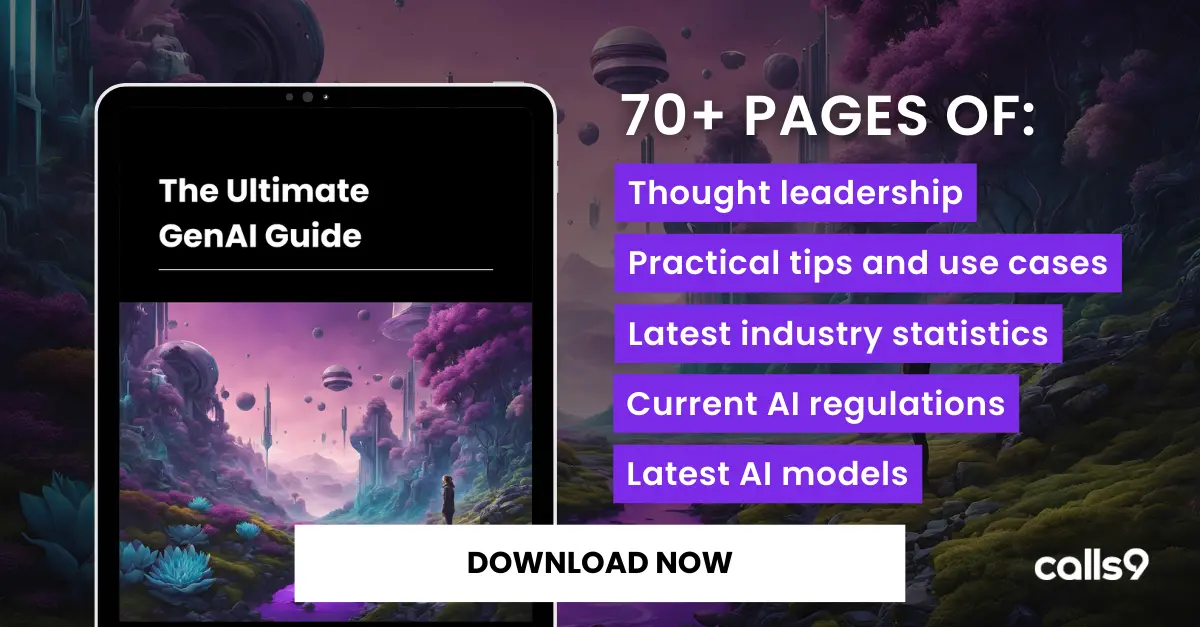
How can I ensure personalisation doesn't cross ethical boundaries?
As the capabilities of generative AI continue to advance, businesses must deploy these technologies with a keen eye on ethics and responsible innovation. Personalisation should empower customers, not exploit them. Ways to safeguard this is:
- Establishing clear guidelines and guardrails around the use of customer data. This includes obtaining explicit consent, providing transparency about data usage, and offering customers control over their personal information.
- Being mindful of potential biases or discriminatory patterns that could be reflected in the data or amplified by AI models.
- Maintaining a human touch in the personalisation process. While GenAI can automate the creation of personalised content, human oversight and review are essential to ensure the final outputs align with the brand's values, messaging, and ethical standards. Consider the example of a financial services firm using generative AI to craft personalised investment recommendations. While the AI can analyse a customer's risk profile, investment goals, and market trends to generate tailored suggestions, a human financial advisor should review the recommendations to validate their suitability. This human-AI collaboration helps maintain the delicate balance between efficiency and empathy.
By striking this balance, businesses can harness the power of generative AI to deliver personalisation at scale while upholding the highest ethical principles. The result is a customer experience that is not only highly relevant and engaging but also built on a foundation of trust and transparency.
Getting Started with End-to-End AI Transformation
Partner with Calls9, a leading Generative AI agency, through our AI Fast Lane programme, designed to identify where AI will give you a strategic advantage and help you rapidly build AI solutions in your organisation. As an AI specialist, we are here to facilitate the development of your AI strategy and solutions within your organisation, guiding you every step of the way:
- Audit your existing AI capabilities
- Create your Generative AI strategy
- Identify Generative AI use cases
- Build and deploy Generative AI solutions
- Testing and continuous improvement
Learn more and book a free AI Consultation
* This articles' cover image is generated by AI