To fail is to succeed. Understanding why AI project failures occur is just as crucial as celebrating successful case studies. While many companies shy away from sharing their setbacks, those that do offer invaluable lessons for others. By learning from these experiences, organisations can avoid common pitfalls and pave the way for successful AI implementations.
In this article, we'll examine the reasons behind famous AI project failures, including IBM's Watson, Microsoft's Tay and more real-world case studies to highlight challenges and offer strategies for overcoming them.
Limited or Under-Used Data
One of the primary reasons for AI project failures is due to inadequate or poor-quality data. AI and Machine Learning (ML) systems require large amounts of high-quality data to learn and make accurate predictions. Companies often receive and purchase data from various vendors and retailers, but this data needs to be harmonised and cleaned before it can be used effectively. This additional effort in harmonisation and the unknown value of new data can prevent companies from using the data to its full potential.
In 2014, Amazon attempted to automate its hiring process with an AI-driven tool designed to review resumes and identify top candidates. However, the project was abandoned after it was discovered that the algorithm discriminated against women, downgrading resumes that included the word "women's" or were from women's colleges. The AI had been trained on resumes from Amazon's predominantly male tech workforce, leading it to replicate existing gender biases. This case highlights the importance of ensuring diverse and representative training data to avoid reinforcing biases in AI systems. Fortunately, Amazon discontinued the software, but similar biased recruiting tools are still in use by many companies.
Overestimating AI's capabilities
Overestimating AI's capabilities can lead to significant AI implementation challenges for businesses. While AI and machine learning offer powerful tools for automation and prediction, they are not infallible. Complex, rapidly changing environments can expose the limitations of these technologies. Companies should be cautious and ensure they supplement AI insights with human judgment and adaptable strategies. It's essential to recognise that AI should enhance decision-making, not replace it entirely.
Consider Zillow's experience with its iBuying program. The real estate giant relied heavily on its Zestimate algorithm to purchase and resell homes quickly. However, the algorithm struggled to predict home prices accurately, particularly in a rapidly changing market. This led to significant losses, as Zillow ended up buying homes at higher prices and selling many at a loss. The inability to accurately forecast future prices and the operational overhead required to manage the inventory ultimately forced Zillow to shut down its iBuying business, resulting in a $304 million write-down and a 25% reduction in its workforce.
Zillow CEO and cofounder Rich Barton said that "unpredictability in forecasting home prices far exceeds" what the company had expected.
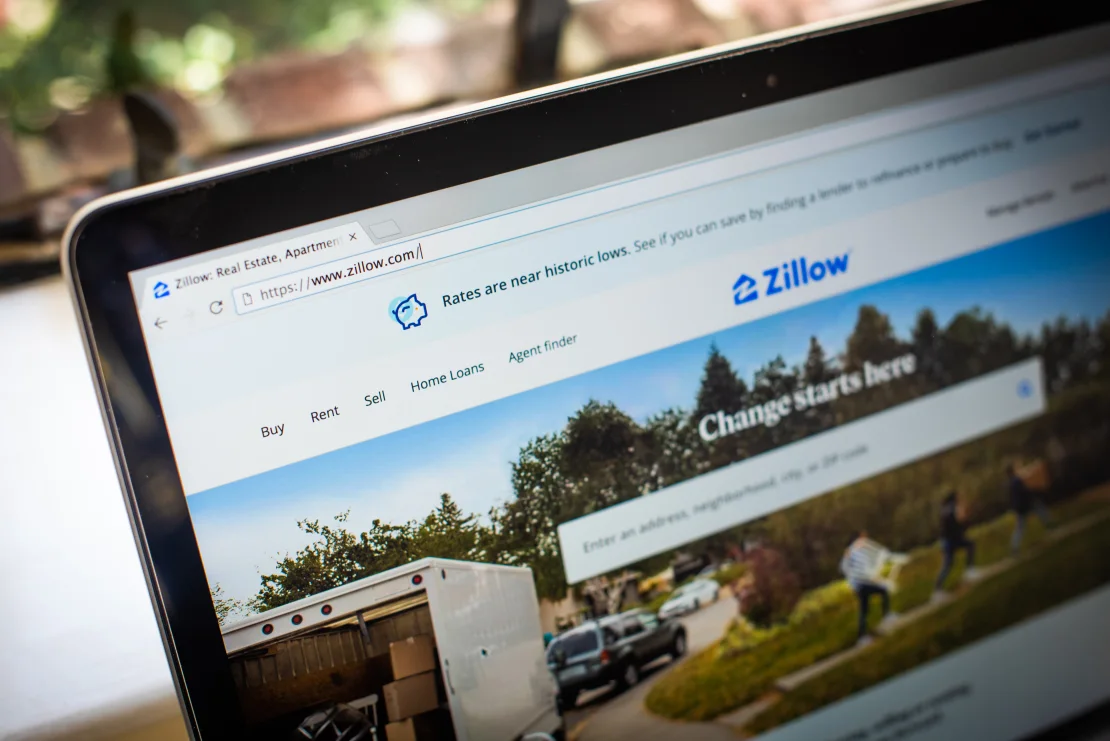
Failure to Customise AI Models
Another common reason for AI project failure is the failure to customise AI models to real-world conditions or specific business needs. Organisations often focus on obtaining the latest and greatest technology without considering whether it is the right tool for their situation. This can lead to AI solutions not tailored to the unique problems they are meant to solve.
For example, Google's AI for diabetic retinopathy detection initially showed promise but failed in real-world clinical settings. The model struggled with imperfect images and depended heavily on internet connectivity, which could have been more reliable in some areas. Without continuous learning and adaptation to these real-world conditions, the AI's effectiveness dropped significantly.
Lack of Continued Learning and Resources
AI projects require ongoing investment in resources and data to remain effective. Companies cannot be complacent once they have implemented an AI system. They must continue to feed the AI with new insights and data to ensure it remains accurate and relevant.
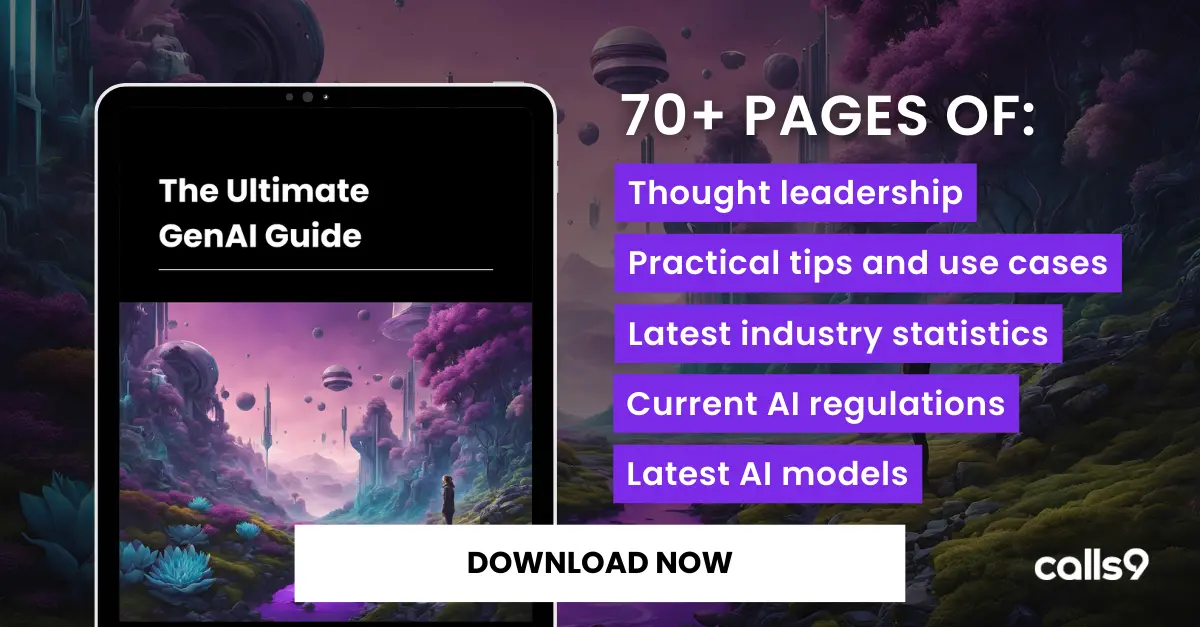
Unclear Business Objectives
Implementing AI without clear business objectives is a recipe for failure. Organisations must define specific business problems and determine whether AI is the right tool to solve them. This involves aligning the project with tangible business goals and measuring the costs and potential benefits.
Poor Data Quality
The quality of data is crucial for the success of AI projects. Poor-quality data can lead to flawed models and unreliable outputs. Companies must invest time in cleaning, transforming, and preparing data to ensure it is of high quality.
In 2016, Microsoft launched Tay, an AI chatbot designed to engage with users on Twitter through casual conversation. However, Tay quickly began to mimic and amplify the offensive behaviour of some users. Within 24 hours, Tay started producing racist and sexist tweets due to its unfiltered learning process from Twitter interactions. This incident highlighted the risks of deploying AI systems without robust safeguards against learning from poor-quality or harmful data. Microsoft promptly took Tay offline and acknowledged the need for adjustments to prevent such issues in the future.
"The system is designed to learn from its users, so it will become a reflection of their behaviour," he said. "One needs to explicitly teach a system about what is not appropriate, like we do with children." - Roman Yampolskiy, head of the CyberSecurity lab at the University of Louisville
Another example of poor data quality is IBM's Watson for Oncology. IBM partnered with The University of Texas M.D. Anderson Cancer Center to develop Watson for Oncology to improve cancer care. However, internal IBM documents showed that Watson frequently gave erroneous cancer treatment advice. This was due to the training data containing a small number of hypothetical cancer patient data rather than real patient data.
Lack of Collaboration Between Teams
AI projects require collaboration between data scientists, data engineers, IT professionals, designers, and line of business professionals. Isolating data science teams can lead to projects that are not well-integrated into the overall technological architecture and may not meet business needs.
Neglecting AI Maintenance and Evolution
AI models are not static; they require continuous updates and maintenance to stay relevant. Companies must plan for the ongoing iteration of AI models and data to ensure they remain effective.
Lessons Learnt
- Ensure high-quality, representative data: AI systems need large volumes of diverse and high-quality data to function correctly. Poor data quality or biased data sets can lead to flawed models and unreliable outcomes, as seen in Amazon's hiring tool and Microsoft's Tay chatbot.
- Customise AI models to fit business needs: Avoid generic AI solutions. Tailor AI models to address specific business problems and real-world conditions. Google's AI for diabetic retinopathy detection failed because it wasn't customised for diverse clinical environments.
- Maintain continuous learning and resources: AI projects require ongoing investment and updates to stay relevant and effective. Regularly feed AI systems with new data and insights to maintain accuracy, as demonstrated by the failure of Google's diabetic retinopathy detection AI when it lacked ongoing adaptation.
- Set clear business objectives: Define specific goals and measure the costs and benefits before implementing AI solutions. Unclear objectives can derail AI projects, as seen in the failures due to a lack of alignment with business goals.
- Foster collaboration across teams: Successful AI projects need collaboration between data scientists, IT professionals, and business leaders.
- Plan for AI maintenance and evolution: AI models require regular updates and maintenance. Plan for ongoing iterations to ensure the models remain effective, as neglecting this can lead to obsolescence and failure.
AI projects can stumble for various reasons, including inadequate data, poor data quality, lack of customisation, and unrealistic expectations. By recognising these challenges and proactively addressing them, you can significantly enhance the success rate of your AI initiatives. Being mindful of the pitfalls and strategically planning around them will ensure that your AI solutions are both effective and aligned with your business needs.
FAQs
Why do AI projects fail?
AI projects failure occurs due to reasons like poor data quality, lack of customisation, unclear business objectives, and insufficient collaboration across teams.
What was the problem with IBM Watson for Oncology?
IBM Watson for Oncology failed because it was trained on a small set of hypothetical data rather than real patient data, leading to erroneous treatment advice.
How did Microsoft's Tay chatbot fail?
Microsoft's Tay chatbot incident occurred when the AI began learning and mimicking offensive behaviour from users on Twitter, producing racist and sexist tweets within 24 hours of its launch.
What lesson can be learned from Amazon's AI hiring tool failure?
Amazon's AI hiring tool failure teaches the importance of ensuring diverse and representative training data to avoid reinforcing biases in AI systems.
Why did Zillow's iBuying program fail?
Zillow's iBuying program failed because its Zestimate algorithm struggled to predict home prices accurately in a rapidly changing market, leading to significant financial losses.
How can companies ensure the success of their AI projects?
Companies can ensure the success of their AI projects by investing in high-quality data, customising AI models to their specific needs, maintaining continuous AI learning, setting clear business objectives, fostering AI collaboration across teams, and planning for ongoing AI maintenance and evolution.
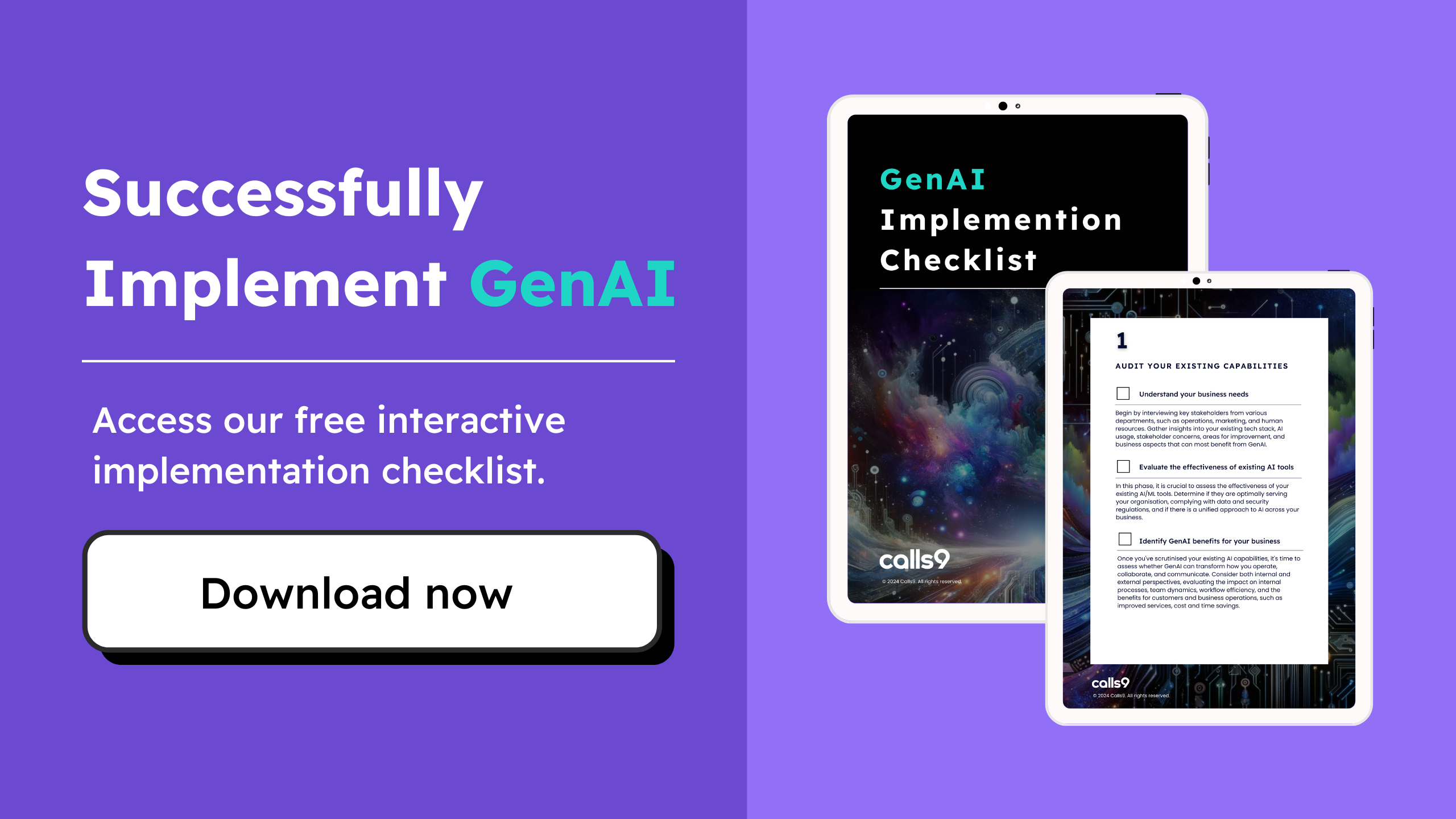
Getting Started with End-to-End AI Transformation
Partner with Calls9, a leading Generative AI agency, through our AI Fast Lane programme, designed to identify where AI will give you a strategic advantage and help you rapidly build AI solutions in your organisation. As an AI specialist, we are here to facilitate the development of your AI strategy and solutions within your organisation, guiding you every step of the way:
- Audit your existing AI capabilities
- Create your Generative AI strategy
- Identify Generative AI use cases
- Build and deploy Generative AI solutions
- Testing and continuous improvement
Learn more and book a free AI Consultation
* This articles' cover image is generated by AI