Generative AI is set to be the next big revolution - transforming the way we live and work. But, let’s pause here—what powers this AI revolution? What gives it the substance to produce reliable results? The answer lies squarely in the data it’s trained on. Like preparing a meal, the end quality of generative AI output is only as good as the ingredients it’s fed. So, what happens when data quality falls short? Poor-quality data can render AI results misleading, untrustworthy, or even damaging, undermining both the utility and credibility of the technology.
In this article, we dive into how data quality impacts generative AI outcomes, explore key metrics for evaluating data quality and provide actionable strategies for ensuring the highest standards of data integrity in generative AI applications. After all, without a focus on data quality, even the most advanced AI models are set up to fail.
The impact of poor data quality on generative AI applications
To grasp the importance of high-quality data, consider two scenarios that reveal just how dramatically data quality can alter the course of AI outcomes. After all, it’s one thing to theorise about data quality; it’s quite another to see its real-world implications.
Scenario 1: The risks of low-quality data in a law firm
Imagine a law firm leveraging generative AI to create case summaries, aiming to speed up research and enhance lawyer efficiency. Yet, the AI model is trained on a dataset with outdated legal precedents, duplicate information and inconsistent formatting. Now, picture a lawyer relying on an AI-generated summary for a high-stakes intellectual property case, only to be provided with outdated interpretations and incomplete references to recent judgments. This misinformation doesn’t just create confusion; it threatens client outcomes and could lead to severe ethical repercussions for the firm. And, more insidiously, it tarnishes the firm’s credibility in the eyes of its clients.
In high-stakes environments like law, data quality is far from a trivial concern. Relying on flawed data yields outputs that are, at best, inaccurate and, at worst, potentially damaging. Without verified and up-to-date information, AI fails to meet the professional standards that clients expect, jeopardising both the firm’s reputation and its client trust.
Scenario 2: The power of high-quality data in a law firm
Contrast that with a law firm whose GenAI is trained on a meticulously curated dataset—one with verified legal documents, recent case precedents, and annotated updates. Here, the AI model produces accurate and insightful case summaries, enhancing lawyers’ preparation with reliable information and up-to-date interpretations. Now, when a lawyer consults the generative AI’s summary, they receive dependable insights that help them advise their clients with confidence, upholding the firm’s standard of excellence.
This scenario showcases the transformative impact of high-quality data on generative AI applications. Armed with consistent and accurate information, GenAI becomes an invaluable resource that amplifies expertise, fosters client trust, and elevates the firm’s reputation. High-quality data empowers AI to deliver meaningful results, underscoring the importance of rigorous data curation.
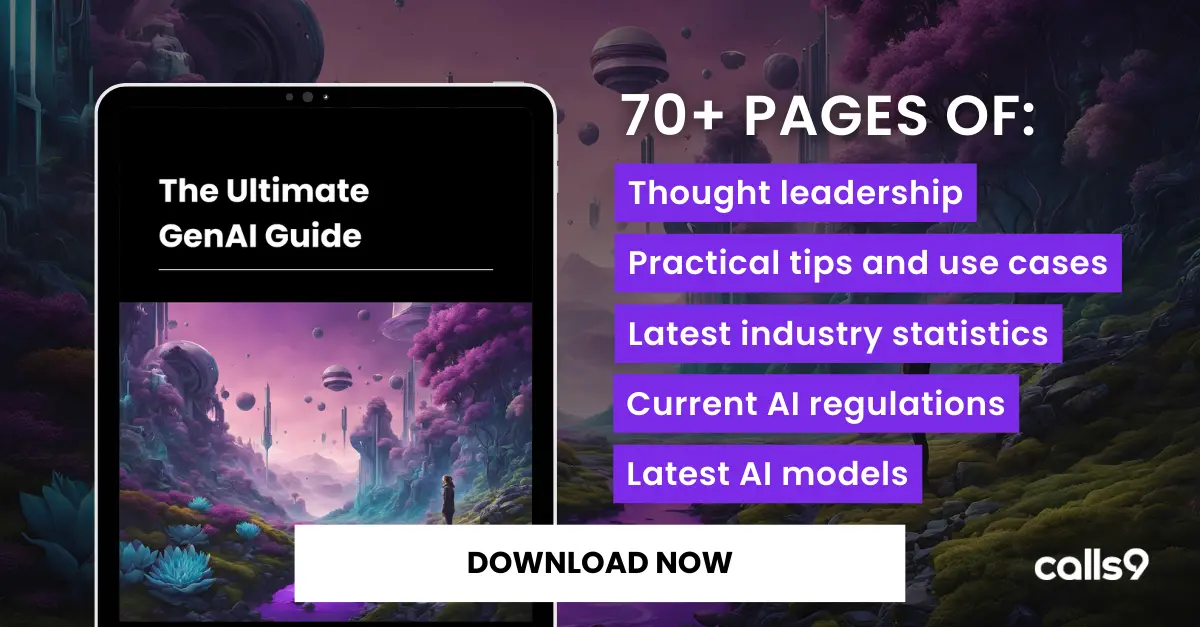
Eight essential metrics for assessing data quality
Now that we’ve seen how poor data can compromise AI, the question becomes: how do we assess data quality to ensure our AI models function effectively? While data quality can seem elusive, breaking it down into measurable metrics can make all the difference. Here are eight critical data quality metrics that organisations should prioritise:
- Accuracy: Does the data accurately reflect real-world events or conditions? Imagine an AI tool designed for customer service—it must rely on accurate feedback data to resolve client issues effectively.
- Completeness: Is any information missing that could affect AI’s contextual understanding? A recommendation engine, for instance, requires a complete view of customer preferences to suggest relevant products accurately.
- Consistency: Is the data uniform across datasets? Discrepancies or contradictions within datasets can lead to unreliable AI outputs, weakening decision-making processes.
- Reliability: Is the data stable and dependable over time? For AI models in predictive maintenance, reliable historical machine data is critical for accurate equipment failure forecasts.
- Relevance: Does the data serve the AI application’s specific purpose? In healthcare, for instance, only relevant patient and treatment data should be included to ensure accurate, usable outputs.
- Timeliness: How current is the data? In time-sensitive applications, like stock trading, outdated data could lead to misinformed decisions and financial losses.
- Validity: Does the data conform to expected formats and values? For AI in diagnostics, patient data must follow standardised clinical formats to ensure consistency in output.
- Uniqueness: Are there redundant entries that could skew AI outputs? Duplicate records in an AI-driven CRM system, for instance, could lead to repeated contacts, frustrating clients and distorting insights.
Strategies for ensuring data quality in generative AI applications
So, we know that data quality is crucial, but what actionable steps can organisations take to safeguard it? Ensuring high data quality requires more than the occasional check; it calls for a strategic approach involving continuous assessment, structured governance, and advanced AI tools.
1. Conduct regular data audits
Data audits are a practical starting point for identifying inaccuracies, biases, and redundancies. Regular audits help organisations detect issues early, preventing them from contaminating AI training. For industries like finance and healthcare, where accuracy is paramount, periodic data audits not only ensure compliance but also enhance model reliability.
2. Implement a data governance framework
Data governance frameworks establish clear protocols for data handling, from entry to processing. By enforcing data standards, governance frameworks minimise inconsistencies and uphold data integrity across all stages. For instance, a framework might require that all external data be standardised before integration, preventing external inconsistencies from entering the system. Robust data governance ensures that every piece of data meets quality standards, promoting long-term reliability.
3. Automate data cleaning and maintenance with generative AI
Generative AI itself can help maintain data quality. AI-powered tools can identify and correct errors, anonymise personal information, and ensure records are current, making them especially valuable for large datasets. Consider the repetitive and laborious task of data cleaning—AI can handle it with precision and speed, correcting errors such as spelling mistakes and outdated metadata. By automating these tasks, organisations save time and ensure the consistency of their data.
.webp)
4. Enhance data with metadata generation and annotation
Metadata provides essential context for data interpretation. By automating metadata generation, organisations enhance searchability, relevance, and organisation, all of which improve data accessibility and usability. For instance, generative AI can tag and annotate customer data, capturing sentiments and enabling more empathetic responses. Metadata transforms raw data into an easily navigable resource, empowering teams to extract meaningful insights faster.
5. Utilise generative AI agents for continuous quality monitoring
Deploying generative AI agents for real-time data monitoring enables organisations to take a proactive approach. Instead of waiting for errors to accumulate, AI agents identify and address data quality issues as they arise, reducing downstream impacts. For sectors managing continuous data flows, like logistics and e-commerce, real-time monitoring ensures data remains consistent, relevant, and reliable, facilitating accurate and timely analytics.
In conclusion, data quality is the foundation upon which effective generative AI applications are built. Without it, even the most sophisticated AI systems are susceptible to producing biased, unusable, or misleading outputs.
For organisations aiming to lead in the age of GenAI, the takeaway is clear: treat data quality as a cornerstone of your AI strategy. Prioritising it is not just a technical necessity; it’s a strategic imperative that can set your business apart, enabling you to drive innovation, make better decisions, and inspire confidence in your AI-driven insights.
Getting Started with End-to-End AI Transformation
Partner with Calls9, a leading Generative AI agency, through our AI Fast Lane programme, designed to identify where AI will give you a strategic advantage and help you rapidly build AI solutions in your organisation. As an AI specialist, we are here to facilitate the development of your AI strategy and solutions within your organisation, guiding you every step of the way:
- Audit your existing AI capabilities
- Create your Generative AI strategy
- Identify Generative AI use cases
- Build and deploy Generative AI solutions
- Testing and continuous improvement
Learn more and book a free AI Consultation
* This articles' cover image is generated by AI