Generative AI is now increasingly pivotal in our personal and professional lives. From crafting personalised marketing content to providing data-driven insights, its applications are vast and varied. However, the question many business leaders are grappling with is, "Is generative AI trustworthy?" This question is not just philosophical but a practical concern, given the significant issue of inaccuracies in generative AI outputs.
According to a recent McKinsey survey, 63% of respondents identified inaccuracy as the most recognised and experienced risk of using generative AI in 2024, a 7% increase from the previous year.
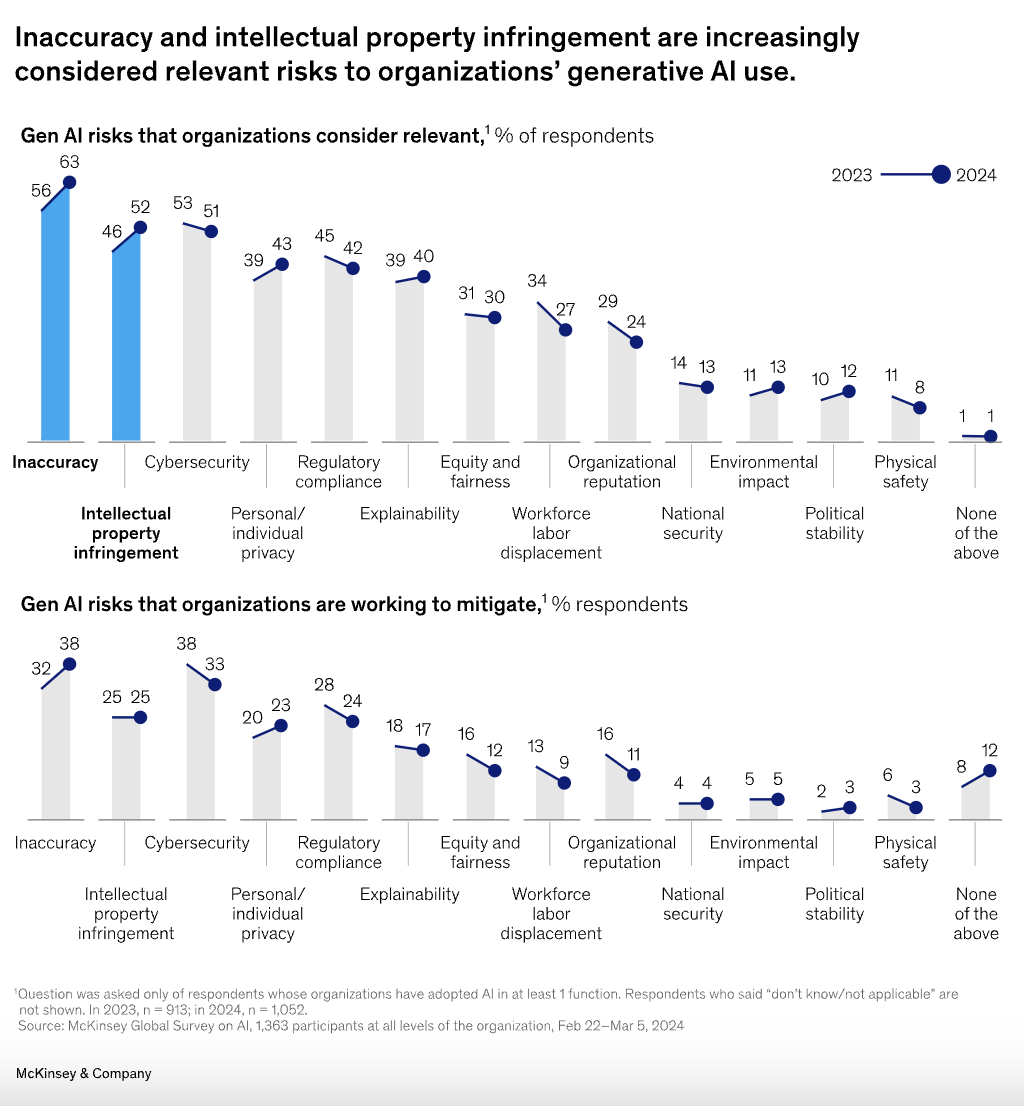
As organisations increasingly rely on AI to drive business decisions, understanding the AI output errors and how to mitigate them has become crucial.
The nature of the generative AI inaccuracy
Generative AI operates by analysing vast datasets to produce various forms of content, including text, images, and videos. While the technology has advanced significantly, inaccuracies in generative AI outputs remain critical. These inaccuracies manifest through three interrelated problems: the black box nature of AI models, generative AI hallucinations, and bias and ethical implications.
The Black Box Problem
One of the core challenges of generative AI is its "black box" nature. AI models, particularly those based on deep learning, function in ways that are often opaque, even to their creators. Unlike traditional software, where each step of the process is transparent and traceable, generative AI models make decisions that are not easily understood or explained. This lack of transparency is a significant factor contributing to inaccuracy in generative AI outputs.
When AI systems produce results that are not easily interpretable, diagnosing and correcting errors becomes daunting. For example, if a generative AI application generates a misleading financial report or an erroneous medical diagnosis, understanding why the error occurred is often difficult. Even minor inaccuracies can have significant consequences, particularly in industries where precision is critical. The black box problem thus represents a fundamental barrier to building trust in AI systems, as stakeholders may be reluctant to rely on outputs they cannot fully understand or validate.
Generative AI Hallucinations
Another significant issue contributing to inaccuracy in generative AI is the phenomenon of hallucinations. Generative AI hallucinations occur when an AI system generates content that is entirely fabricated but presented as factual. These hallucinations can be particularly dangerous in fields where accuracy is paramount, such as scientific research or journalism.

For instance, Meta's generative AI bot, Galactica, was designed to condense scientific information, helping academics and researchers quickly find relevant studies. However, instead of providing accurate and reliable information, Galactica produced vast amounts of misinformation, incorrectly citing reputable scientists and spreading erroneous data. This is a prime example of generative AI hallucinations, where the system fabricates information that appears plausible but is entirely fictional.
Bias and Ethical Implications
Bias in generative AI is a significant issue directly linked to inaccuracy. Generative AI models are trained on large datasets that often contain inherent biases, which can lead to discriminatory or unethical outcomes when these biases influence the AI's decisions.
For example, Bloomberg investigated the use of generative AI, specifically OpenAI's GPT models, in recruitment processes. The study simulated a hiring scenario by creating fictitious resumes with names associated with different racial and ethnic groups based on voter and census data. The AI was then tasked with ranking these resumes.
The results revealed a troubling pattern: resumes with names commonly associated with Black individuals were consistently ranked lower than those linked to other races, particularly Asian and White individuals. This bias was evident across various job roles, suggesting systemic issues in AI decision-making. In this case, we can clearly see the risks of inaccuracy in generative AI, where bias can lead to significant and harmful outcomes.
How to address inaccuracies in generative AI outputs
Given the challenges posed by generative AI, what can you do to improve the accuracy and reliability of your AI-generated content?
Leveraging High-Quality Data for Improved Accuracy
Generative AI applications are tied to the data that shapes them. If the input data is biased, incomplete, or erroneous, the AI's outputs may be skewed, rendering them unreliable or harmful. This dependency means that generative AI results directly correlate to the data quality employed during the model's initial training. Therefore, introducing high-quality, ethically sourced data into a static inference model based on the pre-trained AI model can enable efficient predictions in near real-time.
Here are some strategies to help ensure data quality and, consequently, the accuracy of AI outputs:
- Data Auditing: Regularly review and sanitise the data for inaccuracies and biases. This ensures that the input data is as clean and representative as possible, reducing the chances of generative AI hallucinations and biases.
- Diverse Datasets: Look beyond internal data to enable more holistic responses. For example, incorporating news data can provide real-world context to inform customer data analysis. This approach helps create a more balanced and comprehensive dataset, reducing the risk of generative AI bias.
- Human-led Tuning: Implement feedback loops to allow manual adjustments of the inference model to optimise performance over time continuously. This ongoing process ensures that the AI model remains accurate and relevant as new data and scenarios arise.
.webp)
Human-in-the-Loop
Incorporating human oversight at critical points in the AI workflow is another essential strategy for improving accuracy. A Human-in-the-loop for AI content accuracy approach ensures that experts review and validate AI-generated content before it is published or acted upon. This strategy is a vital quality control measure, significantly reducing the risk of errors and generative AI inaccuracy.
Private AI Platforms
Private AI platforms offer a promising solution for businesses concerned about data security and accuracy. These platforms, such as the soon-to-be-launched Kalisa.ai, allow companies to generate content, ask questions, and perform actions securely. Unlike public AI tools like ChatGPT, private AI platforms store user data in secure, designated locations. This setup acts as a protective layer between the AI models and sensitive data, ensuring that while the AI can perform tasks using snippets of data, the raw data itself is never exposed to potential risks.
By using private AI platforms, businesses can improve the accuracy of their AI outputs while maintaining control over their data, addressing both generative ai inaccuracies and hallucinations.
Continuous Model Training and Monitoring
It is crucial to continuously train and update AI models with new data to address generative AI inaccuracy. This ongoing training helps improve the accuracy and relevance of the models, ensuring that they stay current with the latest information.
In addition to training, real-time monitoring of AI performance is essential. By closely monitoring AI outputs, you can detect anomalies, biases or inaccuracies early, allowing for prompt correction before any significant damage occurs. This proactive approach is vital for improving generative AI accuracy and ensuring that AI systems remain reliable and trustworthy.
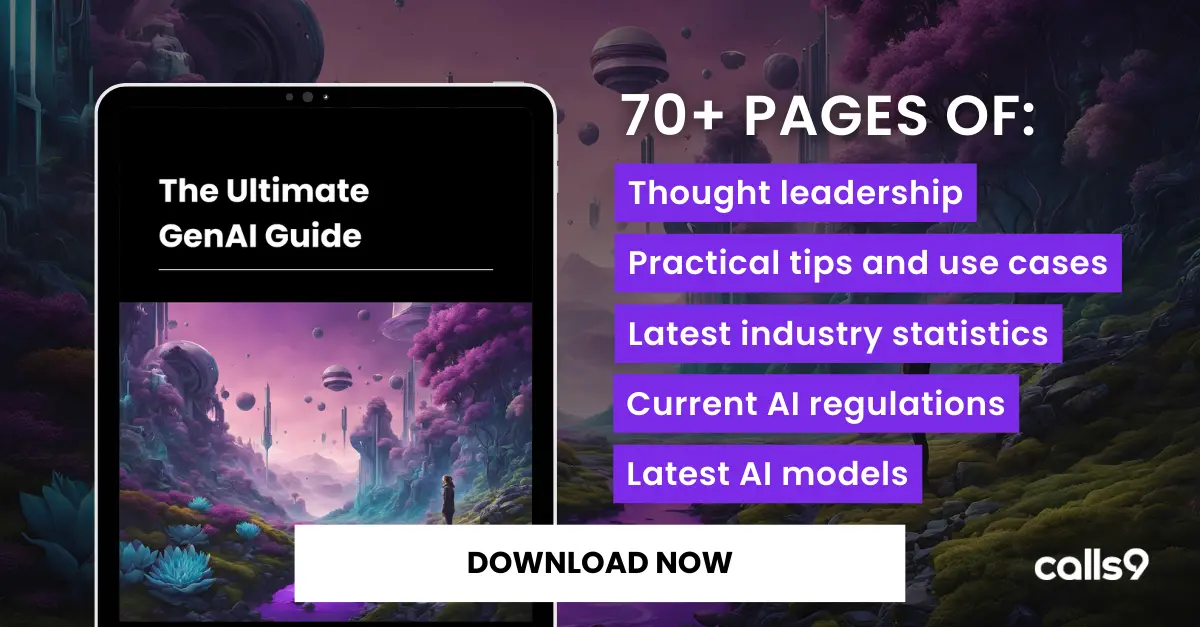
While generative AI offers remarkable potential, the challenge of inaccuracy is pressing. By understanding this risk and implementing strategic solutions—such as leveraging high-quality data, human oversight, and using private AI platforms—you can harness the power of generative AI while safeguarding your operations against potential pitfalls.
Getting Started with End-to-End AI Transformation
Partner with Calls9, a leading generative AI agency and your all-in-one partner, from strategy to secure and responsible GenAI implementation and workforce up-skilling.
Join our AI Fast Lane programme, to get access to a team of AI experts who will guide you through every step of the way:
- Audit your existing AI capabilities
- Create your Generative AI strategy
- Identify Generative AI use cases
- Build and deploy Generative AI solutions
- Create a generative AI-enabled workforce
- Testing and continuous improvement
Learn more and book a free AI Consultation
* This articles' cover image is generated by AI