You’ve likely experimented with AI in some way—whether it’s integrating chatbots, automating processes, or exploring generative AI. But let’s be honest: getting real value from AI is easier said than done. The biggest roadblocks? Accuracy, fairness, and security. AI is only as good as the systems and safeguards in place.
That’s why responsible AI has become a top priority. It’s not just a compliance checkbox—it’s about making AI fair, transparent, and beneficial for users, customers, and society. When done right, responsible AI builds trust. And trust is the ultimate key to adoption, competitive advantage, and long-term success.
Yet, despite its importance, most organisations aren’t fully prepared to implement responsible AI. A recent study found that while 87% of executives see it as a high priority, only 15% feel they’re truly ready to act. So, how do you move from intention to action? This article breaks down what responsible AI really means, the risks of getting it wrong, and the best practices to make AI work for you—responsibly.
What Is Responsible AI and Why Does It Matter?
Responsible AI ensures that artificial intelligence systems operate ethically, fairly, and transparently while minimising risks. It’s about embedding principles of trustworthiness, security, and accountability into AI systems.
The Core Principles of Responsible AI
AI and machine learning models should be built on a strong foundation of trust, fairness, and security. While different organisations may tailor their responsible AI frameworks, most align with the National Institute of Standards and Technology (NIST) principles. These serve as the key pillars of AI trustworthiness and ensure AI systems are ethical, transparent, and resilient.
Here’s what responsible AI should look like:
- Valid and Reliable – AI systems must perform consistently across different scenarios, even in unexpected conditions, without compromising accuracy or functionality.
- Safe – AI should prioritise human safety, protecting lives, property, and the environment from unintended harm.
- Secure and Resilient – AI models must be built to withstand, detect, and recover from cyber threats, including adversarial attacks that could manipulate outputs or compromise data integrity.
- Accountable and Transparent – AI systems must be open and auditable, allowing organisations to trace decisions, identify errors, and fix biases. Developers and businesses should take full responsibility for their AI applications.
- Explainable and Interpretable – Users should understand why and how AI makes decisions. Clear explanations improve trust, allowing businesses to assess whether AI-driven outputs are reliable and fair.
- Privacy-Enhanced – AI must safeguard user data, confidentiality, and individual rights. Responsible AI should be designed with privacy-first principles, ensuring anonymity, security, and user control over personal information.
- Fair with Bias Managed – AI must proactively address bias and discrimination to ensure fair and equitable outcomes. This is challenging, as fairness can vary across cultures and industries, but responsible AI must strive to eliminate unintended prejudice in decision-making.
.webp)
The Risks of Failing to Implement Responsible AI
Ignoring responsible AI doesn’t just expose you to bad PR—it can have real business consequences. Here are some of the biggest risks:
1. Bias and Discrimination
AI models are trained on data, and if that data is skewed or unrepresentative, AI can reinforce existing biases. This can lead to discriminatory hiring practices, unfair loan approvals, and biased law enforcement decisions. A well-known example? An AI-powered hiring tool that unintentionally favoured male candidates over women because it was trained on a dataset dominated by male résumés.
2. Privacy and Security Breaches
AI-powered systems process massive amounts of data—including personal and sensitive information. If not properly secured, this can lead to data leaks, cyberattacks, and regulatory fines. Take the case of Samsung employees accidentally leaking confidential source code into ChatGPT—highlighting the risks of uncontrolled AI use in organisations. To avoid privacy risk, consider encrypting sensitive data, limiting AI access to confidential information, or use secure Generative AI platforms like Kalisa that turn your knowledge and expertise into GenAI use cases like chatbots, automated workflows, client or employee self-service platforms, without compromising on privacy and security.
3. Lack of Explainability and Trust
If customers and regulators can’t understand how your AI makes decisions, trust erodes. AI-generated outcomes that seem random or inconsistent can lead to lawsuits, regulatory crackdowns, and widespread customer distrust. Instead, use explainability frameworks like SHAP or LIME to make AI decisions transparent.
4. Compliance Failures and Legal Risks
Governments worldwide are tightening AI regulations. The EU AI Act, Canada’s AI & Data Act, and various US regulations set strict guidelines on AI use. Failure to comply can lead to hefty fines, legal challenges, and bans on AI applications.
.webp)
5. Operational Disruptions & Shadow AI
Departments often adopt AI tools independently without oversight—leading to inconsistent AI policies, duplicated efforts, and security risks. This “shadow AI” problem creates fragmented, ungoverned AI usage across an organisation.
Best Practices for Implementing Responsible AI
Now that you understand the risks, let’s focus on how to build responsible AI into your organisation. Here’s a step-by-step approach:
1. Establish Clear AI Governance Policies
Start by defining AI ethics guidelines and governance policies. These should align with your company’s values and industry regulations.
- Create an AI ethics committee to oversee responsible AI implementation.
- Develop internal guidelines on fairness, privacy, and explainability.
- Assign accountability to specific teams—who owns AI risk management?
2. Catalog Your AI Models and Data
You can’t control what you can’t see. Many organisations use AI in disconnected and uncoordinated ways. Cataloging your AI models and training data ensures transparency and risk management.
- Conduct an AI inventory to track all AI models in use.
- Regularly audit training data to detect and mitigate biases.
- Ensure critical business data is secured and properly managed.
3. Test AI for Bias, Fairness, and Security
Before deploying AI models, test them for biases, fairness, and security risks.
- Use algorithmic audits to detect biases and unintended outcomes.
- Implement explainability tools to understand why AI makes certain decisions.
- Stress-test AI for vulnerabilities—how does it handle edge cases?
4. Ensure Regulatory Compliance
With global AI regulations evolving, staying compliant is essential.
- Regularly assess your AI against GDPR, the EU AI Act, and other regulations.
- Train employees on responsible AI principles.
- Work with legal and compliance teams to keep AI operations within regulatory boundaries.
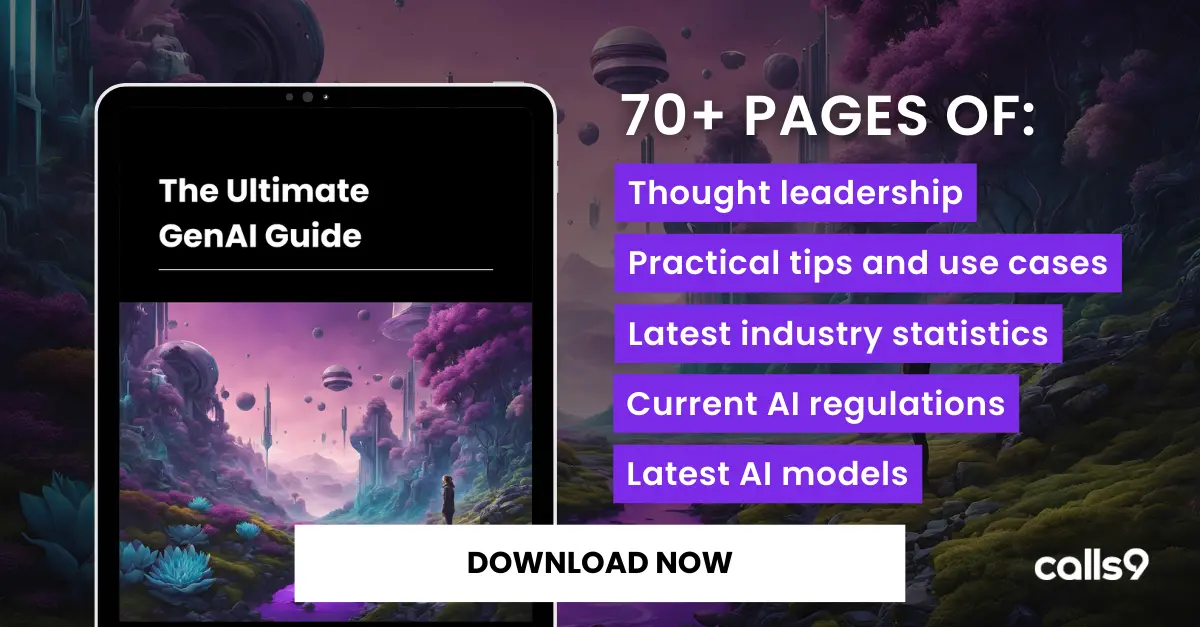
5. Empower Employees with AI Training
AI is only as responsible as the people using it. Employees need the right training to identify risks, use AI effectively, and understand ethical concerns.
- Provide ongoing AI training to employees at all levels.
- Educate teams on bias detection, model interpretability, and responsible AI practices.
- Establish guidelines for AI-assisted decision-making.
6. Monitor AI Performance and Continuously Improve
AI isn’t a set-and-forget technology. Continuous monitoring ensures AI remains accurate, fair, and aligned with your organisation’s goals.
- Implement real-time AI monitoring tools to detect drift and unexpected behaviour.
- Set up feedback loops where users can flag AI errors.
- Regularly update and retrain models with new, unbiased data.
Final Thoughts: Responsible AI as a Competitive Advantage
Here’s the bottom line: responsible AI isn’t just about avoiding risk—it’s about creating value. When customers and employees trust your AI systems, adoption increases, performance improves, and your business gains a long-term competitive edge.
Businesses that prioritise responsible AI today will lead the market tomorrow. The question is—will you be one of them?
If you’re ready to take action, start by auditing your AI practices, setting up strong governance, and ensuring AI fairness, security, and transparency. It’s not just about compliance—it’s about building AI that truly works for people.
Getting Started with End-to-End AI Transformation
Partner with Calls9, a leading Generative AI agency, through our AI Fast Lane programme, designed to identify where AI will give you a strategic advantage and help you rapidly build AI solutions in your organisation. As an AI specialist, we are here to facilitate the development of your AI strategy and solutions within your organisation, guiding you every step of the way:
- Audit your existing AI capabilities
- Create your Generative AI strategy
- Identify Generative AI use cases
- Build and deploy Generative AI solutions
- Testing and continuous improvement
Learn more and book a free AI Consultation
* This articles' cover image is generated by AI